From anatomy of chaos to machine learning-assisted extreme event forecasting
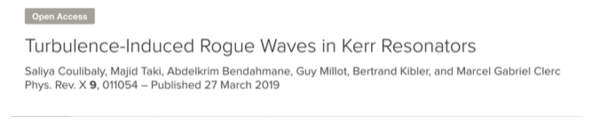
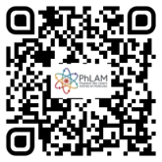
In recent experiments, we have reported the controlled synthesis of a dense soliton gas in deep-water surface gravity waves using the tools of nonlinear spectral theory (IST method) [https://doi.org/10.1103/PhysRevLett.125.264101]. We have evidenced a refraction phenomenon where a tracer soliton experiences an effective velocity change due to its interaction with an optical soliton gas. This effective velocity change has been found to be in good quantitative agreement with the results of the spectral kinetic theory of soliton gas [https://doi.org/10.1103/PhysRevResearch.5.L042002].
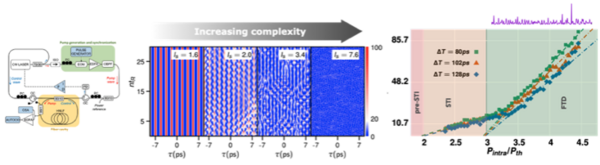
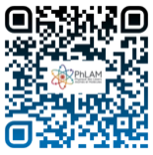
After gaining a comprehensive understanding of the nature and characteristics of complexity within the Kerr frequency comb chaos, we embarked on a collaborative endeavor between two teams at PhLAM to tackle a fundamental challenge in nonlinear dynamics: predicting extreme events such as rogue waves. In this endeavor, we augmented our expertise with a new tool: machine learning, heralding a new paradigm shift. Instead of attempting to predict entire systems, which often necessitate extensive initial state data, we leveraged the synergy between information theory and machine learning [Chaos, Solitons & Fractals 160 (2022)]. This innovative approach identifies early warning signals for significant deviations, enhancing prediction accuracy while mitigating noise interference.
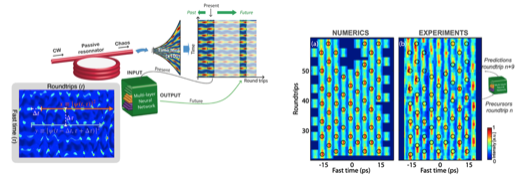
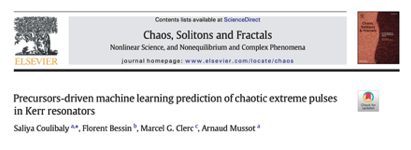
1) An international reference in topological photonics
2) Ultrafast observation and control of particle accelerators
3) Soliton gases in Optics and in Hydrodynamics (SOGOOD ANR Project)
5) New states of light : from quantum to nonlinear regime
6) Data-driven modeling of cell metabolic response to oxidative stress
7) Novel opto-fluidic drug delivery system for efficient cellular transfection